About me
I am a student of Hong Kong University of Science and Technology (Guangzhou), majoring in Robotics and Autonomous Systems, in Transportation Behavioral Psychology and Safety Lab (TBPS).
Research interests include Human-computer Interaction in Autonomous Driving, Human Factors, Accessibility Design, Social Computing and Network Analysis.
My previous work was in ByteDance and Netease Interactive Entertainment as a product manager, designing dealer middle-end systems, and customer service chatbot, Identifying risks and abnormal transactions through data.
What i'm doing
-
Lightweight Crowd Counting
We proposed a lightweight model for accurate crowd counting, maintaining high efficiency. By compressing the CNN and Transformer models simultaneously, we transferred knowledge from a SOTA teacher model, achieving excellent performance. Experimental results confirmed the success of our knowledge distillation methods, reducing the parameter size to less than one-tenth of the teacher model while maintaining comparable accuracy.
-
Improving Safety for Novice Drivers in SAE Level-2 Vehicles: Integrating ADAS-limitation and Hazard Perception Training
ADAS are increasingly common, especially among novice drivers. Most training focuses on system limitations, but effective takeover also requires hazard detection. This study tested whether combined ADAS limitation and hazard perception (AD+HP) training improves takeover performance compared to AD or HP training alone. In a simulator study with 32 participants, AD+HP training led to earlier takeovers, quicker hazard recognition, and better safety outcomes. Though trust in ADAS increased post-training, it dropped after testing, with workload higher in the AD+HP group. The findings suggest AD+HP training could be more effective and shape future training policies for ADAS users.
-
Evaluating Large Language Models on Academic Literature Understanding and Review: An Empirical Study among Early-stage Scholars
The rapid rise of large language models (LLMs) enables LLM-based academic tools, but little research has evaluated their use in scholarly tasks. In this study, 48 early-stage scholars performed core academic activities (paper reading and literature reviews) under varying time pressures, after receiving training on LLM limitations and capabilities. Performance data and interviews revealed how time pressure, task type, and training affected outcomes, as well as scholars' perceptions and concerns about LLMs. The results offer insights for optimizing LLM tools for academic work.
-
Classification of driver cognitive load in conditionally automated driving: Utilizing ECG-based spectrogram with light-weight neural network
As conditionally automated driving enabled non-driving tasks, monitoring cognitive load became crucial for safe takeovers. Estimating cognitive load was challenging due to feature representation and individual differences. This study proposed a SENet-based deep learning method to classify cognitive load using ECG spectrograms, bypassing manual feature extraction. The method achieved 96.76% accuracy in within-subject and 71.50% in across-subject evaluations, demonstrating its feasibility for detecting driver cognitive load.
-
Marvel Network Analysis
Network modeling, visualization, and analysis of comic book characters in the Marvel Universe.
-
Two-way Intergenerational Communication VR Design
Using VR to increase the frequency and improve the quality of communication between the elderly and their children.
Testimonials
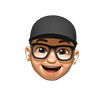